Oftentimes researchers want to compare two medications—an existing medication to a newly approved medication, for example—to understand which is more effective in treating or preventing disease. To do this in a real-world data (RWD) setting, we need to separate the treatment effect from the effects of confounding factors, which are the factors that impact the medication a patient receives and their risk of having the outcome of interest.
While epidemiologists have several ways to deal with confounding, one set of methods, which includes propensity score matching and weighting, focuses on creating baseline comparability between the two treatment groups on potential confounding factors.
Below, Trisha Prince, MPH, Associate Director of Science at Aetion, explains three different propensity score weighting methods, when and how to use each, and how using an RWE platform can help researchers easily test and apply the most appropriate analytical method to their study.
Responses have been edited for clarity and length.
Q: What is propensity score weighting?
A: We use propensity score methods in comparative studies to control for confounding variables before assessing the impact of a treatment on outcomes. These methods help us to isolate the effect of a treatment from other differences that may exist between treatment and comparison groups. That way, we can be certain that differences in outcome between the two groups came as a result of taking a particular treatment.
There are a few different ways you can use propensity scores in the assessment of treatment effects. One of the most common methods is propensity score matching. But depending on your study, it might be more appropriate to use propensity score weighting instead.
Propensity score weighting assigns patients different “weights”—weighting them up or down to make the patients in the treatment group and the comparison group more similar to each other. One of the advantages of using propensity score weighting, as opposed to matching, is that you’re able to include all patients; none of the patients are excluded because they can’t be matched to a patient in the other treatment arm. Including all the patients is especially important when you have small sample sizes. Propensity score weighting allows you to leverage information from all patients included in your sample.
My colleague Adina Estrin outlined the differences between propensity score matching and weighting in the diagrams below:
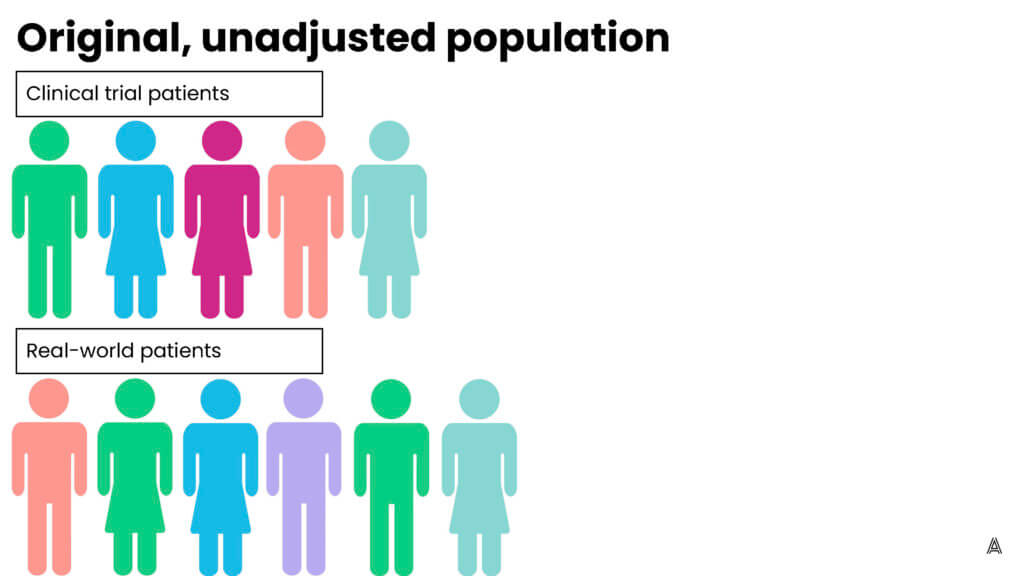
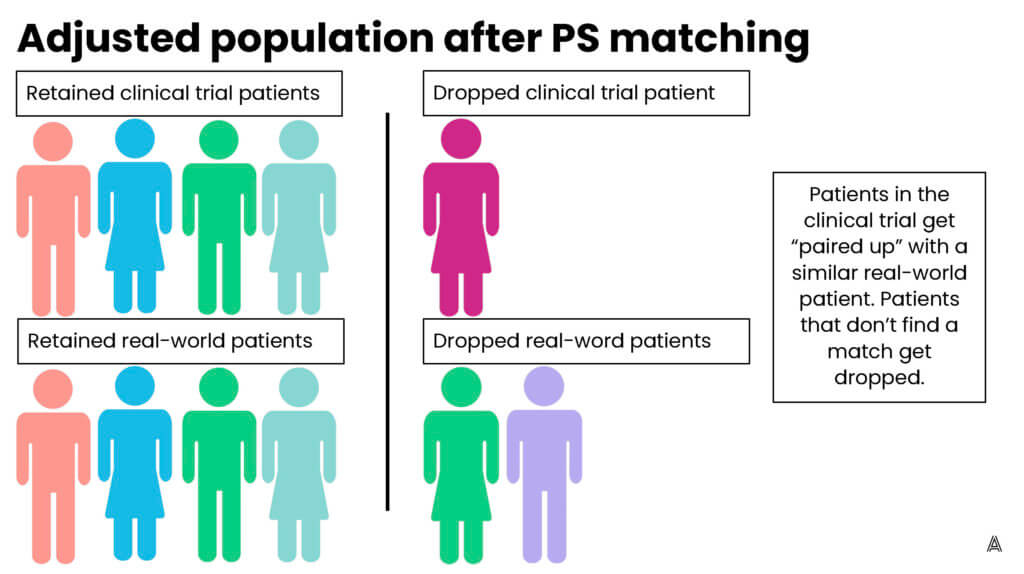
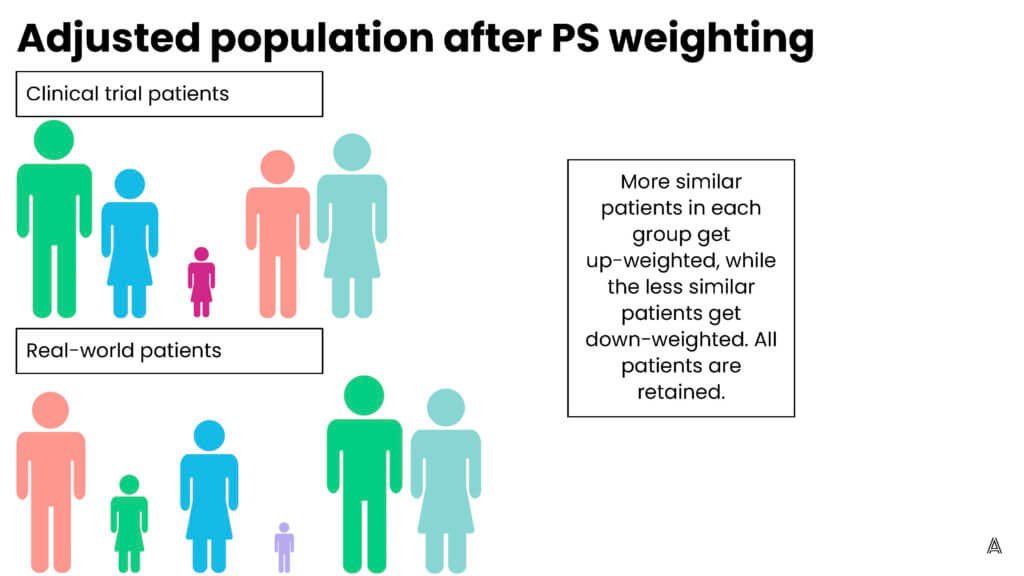
Q: What are the different weighting methods that epidemiologists use?
A: There are a lot of different propensity score weighting methods, but the most common ones that are used in RWE studies are (1) inverse probability of treatment weighting (IPTW), (2) standardized mortality or morbidity ratio (SMR) weighting, and (3) overlap weighting.
Q: When would you use each of these methods?
A: There are different strengths and weaknesses associated with each of the weighting methods. To evaluate between these different weighting approaches, you first need to understand the target population in which you’re looking to estimate the treatment effect. If you want to estimate the average treatment effect assuming that every patient (both treated and comparison group patients) in the population would otherwise be offered the treatment, which is known as the average treatment effect (ATE), you would use IPTW.
SMR weighting and overlap weighting allow you to estimate the treatment effect in different populations. SMR weighting allows you to estimate the ATE among those who were treated; overlap weighting will estimate the treatment effect among patients who are most similar to one another. One of the advantages to using SMR weighting as opposed to IPTW and overlap weighting is that it allows you to preserve the results of one of the study arms. Essentially, it makes patients in one treatment arm similar to those in another arm. This is especially useful for external control arms (ECAs), where the goal is to preserve clinical trial arm results and make the ECA generated from a real-world population more similar to the clinical trial population. So rather than making the ECA and clinical trial populations similar to each other, it makes the ECA look like the clinical trial population.
Overlap weighting helps overcome some of the limitations of IPTW and SMR weighting. One of the major limitations to both IPTW and SMR weighting is that they’re subject to extreme weights. This occurs when a researcher performs propensity score weighting and certain patients are “up-weighted” very highly such that a single patient can count as up to 10 patients in our population—10 times their original weight. In contrast, overlap weights are bound between zero and one, so you don’t have to worry about extreme weights.
Another issue with IPTW and SMR weighting is that even after you apply the propensity score weighting, it’s possible to have residual confounding in your populations. Overlap weighting ensures that all of the confounders in your propensity score model will be perfectly balanced between the two arms of your study.
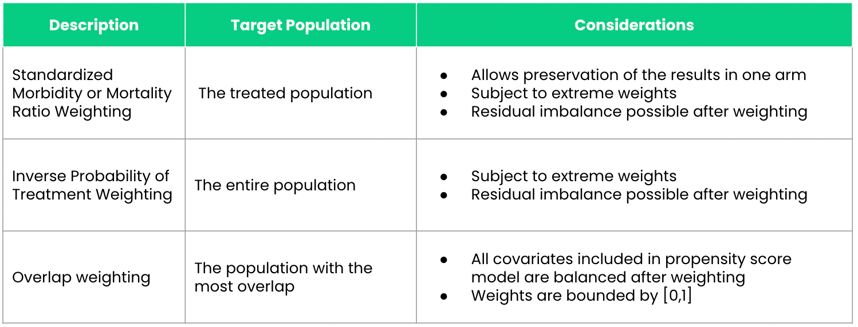
Q: How does using an RWE platform enable best practices for researchers using those weighting techniques?
A: One of the advantages of using a platform, such as the Aetion Evidence Platform® (AEP), is that you’re able to evaluate the different methods of weighting and matching. As explained, there are strengths and limitations associated with each of these methods. A rapid-analytics platform allows you to easily perform exploratory analyses where you apply some or all of the methods and look at diagnostics such as baseline comparability between the two groups to inform a decision about the best methods to use. The selected method is then used in the inferential analysis where outcomes are evaluated.
For example, perhaps it’s important for this study to preserve the trial population. SMR weighting may be useful; however, if you see extreme weights, SMR weighting may not be the best method. The platform allows you to apply the methods, assess the baseline comparability, then choose which method is best before you look at the outcomes in the population. This iterative approach helps you understand whether you’ve achieved the balance you meant to achieve, and whether patients look comparable, before you move on to look at outcomes to assess treatment effects.
Another advantage of using a platform versus line programming relates to the nuances of these weighting methods. A platform allows you to consistently apply the same complex methods across different studies. When you’re doing overlap weighting in one project, you know that the platform will apply the same methods to the next project rather than having two different programmers applying slightly different codes and thus inadvertently different methods.
Q: Can you provide an example of a time that you used weighting methods in an RWE study? How did they help ensure that you achieved reliable results?
A: As I mentioned before, we often use SMR weighting for external controls. In one rare oncology project using ECAs derived from RWD compared to treated trial patients, we had very small sample sizes. We knew that propensity score matching was out of the question because we wanted to leverage all of the clinical trial information that we had available, as well as all of the available RWD.
We used an iterative process of running exploratory analyses on the AEP to select the right propensity score weighting method. As expected, when we applied propensity score matching some clinical trial patients were unable to be matched. We then moved on to assess SMR weighting and evaluated baseline comparability with SMR weighting to understand if the RWD and trial populations were comparable.
We saw that the RWD arm and clinical trial arm were comparable after weighting , in terms of the confounders that we had included in our model. This baseline comparability between the two groups allowed us to be certain that the treatment effect we saw was truly a result of the treatment given in the clinical trial, and not because the real-world population was different than the clinical trial population.
Special thanks to Mandy Patrick and Pattra Mattox for their support on this piece.